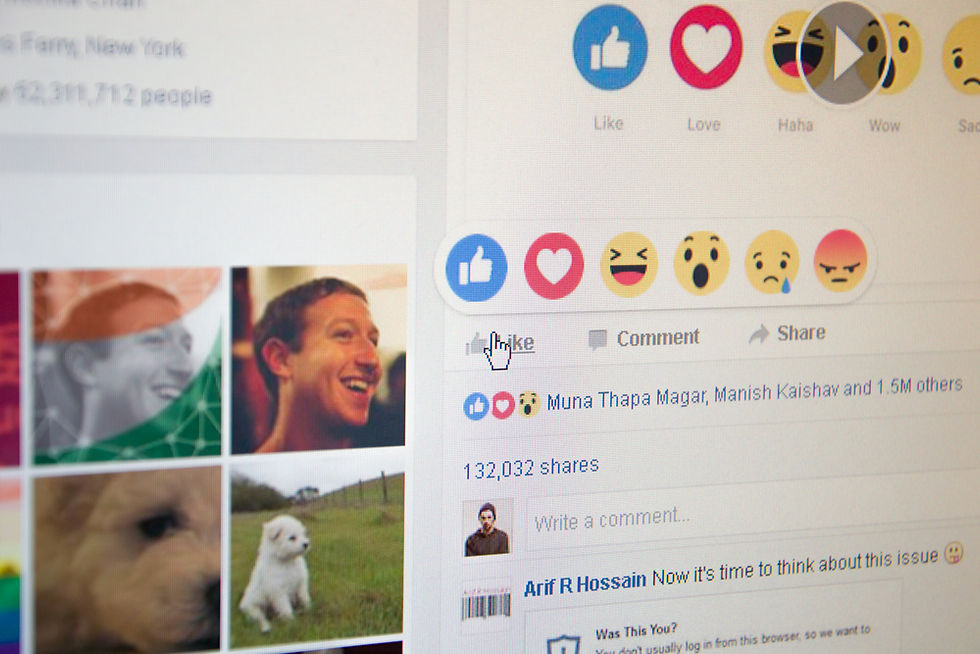
Let's break down the analysis and recommendations for this situation step by step:
1) Clarifying Questions:
Is this change consistent across all user segments or specific to certain demographics?
Did the overall user engagement (likes + comments) increase or decrease?
Are there any recent feature updates or changes that could have influenced these metrics?
Is the drop in comments statistically significant?
Are there any external factors (e.g., events, holidays) that could have impacted user behavior?
Have there been any changes in the way users interact with posts or content?
2) Product Description and Objective:
Facebook is a social media platform that allows users to connect, share content, and engage with each other through posts, likes, comments, and other interactions. The objective is to maintain and enhance user engagement while ensuring a positive user experience.
3) Hypotheses:
Competing Features: The introduction of new features (e.g., reactions, stories) might be diverting user attention from comments to other forms of engagement.
Visibility of Likes: Increased visibility of likes is encouraging users to engage with posts using likes rather than comments.
Algorithm Changes: Recent algorithm updates might be prioritizing posts that receive more likes, leading to decreased emphasis on comments.
Content Type Shift: Users might be sharing more visual or concise content that is more conducive to likes than comments.
Quality of Comments: A decline in comment quality or relevant discussions might discourage users from commenting.
Negative Sentiment: A surge in negative sentiment could lead users to avoid commenting while still engaging with likes.
User Fatigue: Users might be experiencing "comment fatigue" and prefer quick likes over more time-consuming comments.
4) Operationalizing Hypotheses:
Competing Features: Analyze the adoption rate of new features and their impact on user engagement. Compare engagement metrics between posts with and without new features.
Visibility of Likes: Analyze the distribution of likes and comments per post. Visualize the ratio of likes to comments over time.
Algorithm Changes: Examine engagement patterns before and after the algorithm update. Use A/B testing to assess the impact of different algorithms on likes and comments.
Content Type Shift: Categorize posts based on content type (e.g., text, images, videos). Compare engagement trends across different content types.
Quality of Comments: Implement sentiment analysis to evaluate comment quality and sentiment over time. Compare the sentiment of comments before and after the observed change.
Negative Sentiment: Analyze comments for keywords and sentiment associated with negativity. Investigate if negative sentiment has increased recently.
User Fatigue: Study user engagement patterns over time. Assess the average time spent on the platform and interaction frequency per user.
5) Conclusion and Recommendation:
Based on the analysis, it appears that increased visibility of likes and potential algorithm changes are likely contributing factors to the observed trends. To drive balanced engagement and promote meaningful interactions, it is recommended to:
Monitor and fine-tune the algorithm to ensure a healthy mix of likes and comments.
Encourage users to leave thoughtful comments through prompts or rewards.
Provide clearer guidelines for meaningful comments to enhance comment quality.
Remember, continuous monitoring and iterative testing of hypotheses will be crucial to adapt and optimize the platform's features and algorithms for sustained user engagement and satisfaction.